The Rise of Digital Colleagues: How Agentic AI is Transforming Business Process Automation
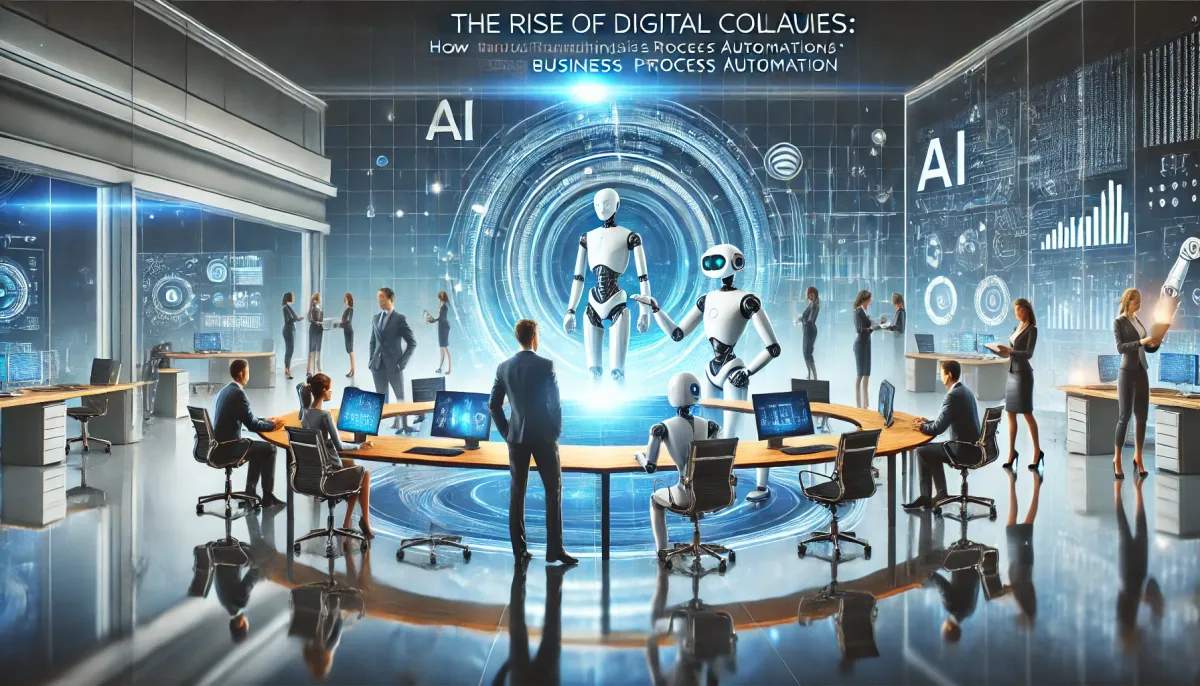
In 2025, enterprises are moving beyond traditional automation as AI evolves from simple assistant to autonomous agent. This transformation is redefining how organizations approach complex processes, creating new opportunities for efficiency while challenging conventional workforce models. Is your organization ready to collaborate with its new digital colleagues?
The Evolution: From Tools to Autonomous Agents
The business automation landscape is undergoing a profound transformation from rule-based systems to autonomous AI agents capable of reasoning, learning, and making decisions.
"With AI agents on the horizon, there will be a significant number of business processes that will be a much better fit for AI than we have previously seen," notes Gary Bailey, CIO at Phillips Edison & Co.
Traditional automation approaches like RPA excel at performing structured, repetitive tasks but struggle with exceptions or scenarios requiring judgment. Agentic AI represents the next evolutionary leap, with capabilities to:
- Process information contextually
- Make decisions based on complex criteria
- Execute multi-step tasks autonomously
- Learn and adapt from experience
- Collaborate with humans and other AI systems
This progression from passive tools to active agents transforms business process automation from tactical improvement to strategic reimagining of operations.
End-to-End Process Automation: Beyond Simple Tasks
The most significant shift is agentic AI's ability to manage end-to-end processes rather than just discrete tasks.
Traditional automation excels at handling individual steps but typically requires human intervention at decision points. The result is a fragmented process with automation islands connected by manual handoffs.
Agentic AI fundamentally changes this paradigm by enabling:
Comprehensive Process Oversight
AI agents can oversee entire processes from start to finish. For example, in insurance claims processing, an agent can intake documents, extract information, assess coverage, determine validity, calculate payouts, initiate payments, generate communications, and document everything for compliance.
A large Dutch insurance provider now automates approximately 90% of individual automobile claims using AI agents, enabling human adjusters to focus exclusively on complex cases.
Complex Decision-Making Capabilities
Unlike rule-based systems, agentic AI navigates ambiguity and makes nuanced decisions. Saket Srivastava, CIO at Asana, explains: "These workflows allow AI agents to handle repetitive, manual tasks like triaging project requests, drafting briefs, or assigning work, significantly reducing busy work."
This reasoning capability—not just following predefined rules—represents a fundamental shift, allowing evaluation of multiple factors, weighing competing priorities, and making decisions that previously required human judgment.
Adaptive Response to Changing Conditions
Agentic AI systems respond adaptively to changing circumstances. As Victor Kushch, Co-founder and CTO of Fairmarkit, notes: "Unlike previous AI models, which functioned as reactive tools, these agents autonomously drive workflows, applying reasoning and adaptive decision-making at every stage."
This adaptability means processes remain resilient even when conditions change or exceptions arise.
Digital Colleagues: AI Working Alongside Humans
The emergence of AI agents is creating a new paradigm of human-machine collaboration best understood as "digital colleagues" rather than simply tools.
"AI coworkers such as virtual assistants, agents, copilots, and autonomous workplace assistants continuously learn to analyze, interpret, and take action on data in context," explains Forrester Research.
In this evolving workplace, AI agents take on expanded responsibilities:
Independent Problem Solving
Digital colleagues identify issues, develop solutions, and implement fixes with minimal human intervention. For example, in IT operations, AI agents can detect system anomalies, diagnose root causes, and execute remediation steps—all before a human would typically become aware of the problem.
Specialized Expertise Augmentation
AI agents encapsulate specialized knowledge and apply it consistently. PepsiCo uses AI agents in talent recruiting to rank candidates based on job requirements, enabling human recruiters to focus on evaluating the best-matched candidates.
Cross-Functional Coordination
Agentic AI excels at orchestrating work across traditionally siloed departments. Priya Iragavarapu, VP of Data Science and Analytics at AArete, notes this advantage: "When a business process spans several siloed teams, it is best to build an AI agent that can be cross-trained for this cross-functional expertise."
For example, in order-to-cash processes, AI agents can integrate data from sales, finance, and logistics teams, providing a unified view to identify bottlenecks and assist in reconciliation tasks.
Process Redesign: Reimagining Workflows for AI Collaboration
Successfully implementing agentic AI requires fundamentally redesigning workflows to leverage the unique capabilities of these autonomous systems.
"A successful agentic AI strategy starts with a clear definition of what the AI agents are meant to achieve," advises Prashant Kelker, Chief Strategy Officer at ISG. "Agentic AI needs a mission."
This redesign process involves several critical considerations:
Identifying Suitable Processes
According to Deloitte, 25% of companies using generative AI will launch agentic AI pilots in 2025, growing to 50% by 2027. Processes ideal for agentic AI typically share specific characteristics:
- High-value, repetitive workflows with clear objectives - Processes that follow consistent patterns but deliver significant business value
- Processes spanning multiple systems or departments - As Asana's Srivastava explains, "When processes span multiple teams and require significant coordination, they can benefit from AI's ability to act as an orchestrator"
- Data-rich environments with quality information - "To derive tangible value from agentic AI, companies need high-quality data," Srivastava emphasizes
- Processes with hybrid automated/manual components - AArete's Iragavarapu highlights that "Agentic AI is best applicable for business processes that have dual approaches of programmatic and manual tasks"
Redesigning Control Flows
Effective process redesign must reconsider how control flows between human and AI participants. Rather than simply automating individual steps, organizations should reimagine workflows based on the distinct strengths of each participant.
For example, in customer service operations:
- AI agents handle initial contact, routine inquiries, and data gathering
- Human agents focus on complex problem-solving and relationship building
- AI agents assist humans by retrieving relevant information and suggesting solutions
- Process performance data is continuously analyzed to refine the division of responsibilities
Building Feedback Loops
Processes must incorporate feedback mechanisms that enable continuous improvement through:
- Clear metrics for success that both humans and AI understand
- Mechanisms for humans to provide feedback on AI decisions
- Automated performance monitoring that identifies improvement opportunities
- Regular review cycles to assess and refine the human-AI collaboration model
Competitive Differentiation: Strategic Advantage Through Agentic AI
Beyond operational efficiency, agentic AI creates strategic competitive advantages through:
Unprecedented Scalability
AI agents enable organizations to scale operations without proportional increases in headcount, responding to market opportunities more rapidly than competitors relying on traditional workforce models.
A global e-commerce company deployed AI agents to manage product listings across multiple international markets, expanding their catalog by over 200% in six months without adding staff.
Enhanced Customer Experiences
AI agents deliver personalized, responsive customer experiences at scale by maintaining context across interactions, accessing comprehensive customer data, and making informed decisions.
Forrester notes that AI agents "empower self-directed buying, acting as a personalized concierge that understands, guides, and supports buyers and customers across delivery channels."
Operational Resilience
Organizations with well-implemented agentic AI demonstrate greater resilience to disruptions, maintaining operational continuity through their digital workforce.
As Kushch observes, "Businesses that treat AI as a tactical tool will quickly fall behind those that build AI into the fabric of how decisions are made."
Implementation Challenges and Considerations
Organizations face significant challenges in implementation that must be addressed:
Data Foundation Requirements
Quality data is essential for effective agentic AI. Organizations must invest in:
- Data quality initiatives to ensure accuracy and relevance
- Integration capabilities that provide AI agents with comprehensive context
- Governance frameworks that maintain data integrity
- Feedback mechanisms that continuously improve data quality
Governance and Oversight
Autonomous AI agents require robust governance frameworks that establish:
- Clear policies on which decisions AI agents can make independently
- Escalation paths for scenarios requiring human judgment
- Monitoring systems to ensure AI decisions align with organizational values
- Audit capabilities that provide transparency into AI decision processes
Change Management Challenges
Organizational resistance is often the most significant barrier. Effective implementation requires:
- Executive sponsorship with a compelling vision
- Transparent communication about how AI will augment rather than replace humans
- Training programs for effective human-AI collaboration
- Redesigned incentive structures that reward collaboration
Looking Ahead: The Future of Work with Agentic AI
Several emerging trends suggest how human-AI collaboration will develop:
Specialized AI Workforces
Organizations are developing specialized AI workforces with agents designed for specific business functions, similar to how human workforces include specialists with distinct expertise.
AI Orchestration Platforms
As AI agents proliferate, platforms for coordinating multiple specialized agents become increasingly important. Forrester notes, "With multiple AI agents in play, orchestration will be critical. They need to cooperate with one another to plan and take action on multistep processes."
Hybrid Teams as the New Normal
Successful organizations are moving toward models where teams comprise both human and AI members, each contributing their distinct capabilities to achieve shared objectives.
"By 2025, the conversation will no longer be about whether AI can automate tasks—it will be about how businesses structure themselves around AI-driven decision-making," predicts Kushch.
Conclusion: Preparing for the Agentic AI Future
Organizations preparing for this future should:
- Assess process portfolios to identify high-potential opportunities
- Invest in data quality as the foundation for effective AI agents
- Develop governance frameworks that enable appropriate autonomy
- Create change management strategies for human-AI collaboration
- Experiment with pilot implementations to build organizational capability
As Gary Bailey notes, "We are in the midst of an extremely opportunistic window." The question is no longer whether AI will transform business process automation, but which organizations will lead this transformation.