Taming the Data Tsunami: Strategic Approaches to Data Management and Analytics Challenges in 2025
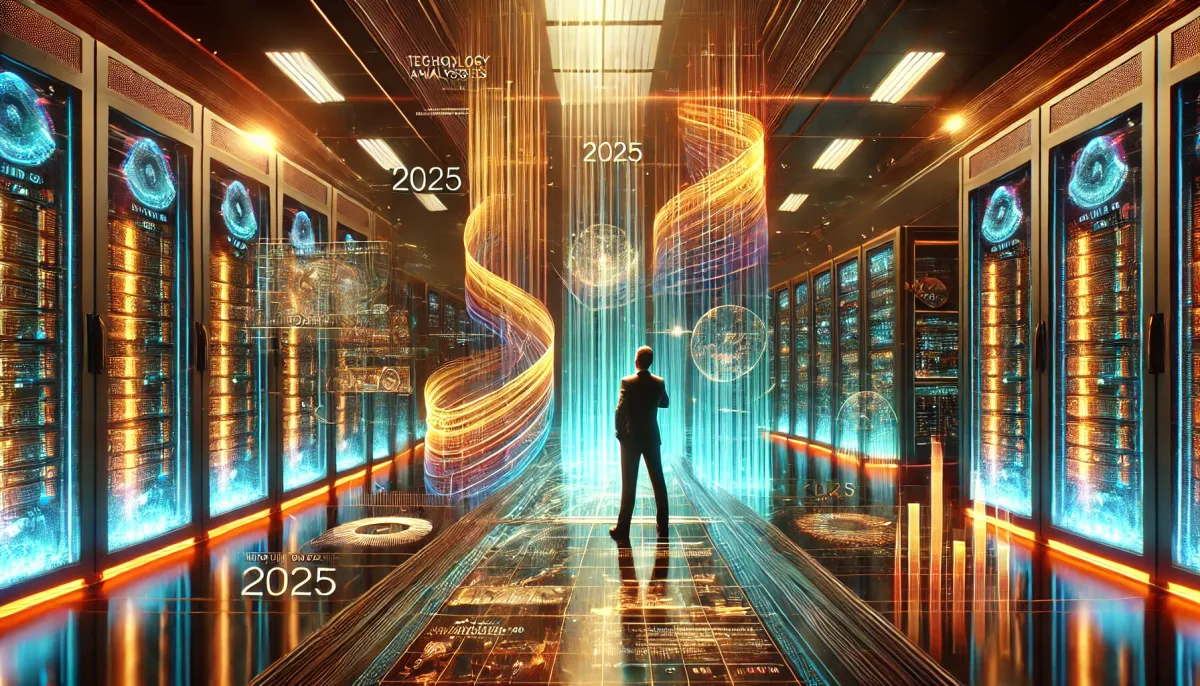
As organizations navigate the increasingly data-centric business landscape of 2025, the volume, variety, and velocity of data continue to accelerate at an unprecedented pace. For technology leaders, the ability to effectively harness this data deluge has become a fundamental differentiator between market leaders and laggards. This article explores the critical challenges facing CTOs and CIOs in data management and analytics, offering strategic approaches to transform these challenges into competitive advantages.
The Expanding Data Universe: Scale and Complexity
The exponential growth of data shows no signs of slowing. According to industry analysts, the global data-sphere is projected to reach 175 zettabytes by 2025, representing a staggering 50% compound annual growth rate over the preceding five years. This growth is driven by the proliferation of connected devices, the widespread adoption of cloud services, and the increasing digitization of business processes.
For enterprise technology leaders, this data explosion creates both immense opportunities and significant challenges. Organizations that can effectively manage and derive value from their data assets gain powerful competitive advantages, while those that struggle with data management risk being overwhelmed by the sheer scale and complexity of their information assets.
"The year 2025 will see more mandates than ever before when it comes to the management of valuable unstructured data assets," notes a recent report from Komprise. This increasing focus on data as a strategic asset requires technology leaders to develop sophisticated approaches to address four critical data management and analytics challenges.
Challenge 1: Unstructured Data Growth - Taming the Wild West
The Challenge
Unstructured data—including documents, images, videos, social media posts, emails, and sensor data—now constitutes more than 80% of all enterprise data and is growing at a rate three times faster than structured data. This explosive growth presents several critical challenges:
- Storage and Infrastructure Demands: Unstructured data consumes vast amounts of storage resources, often leading to escalating costs and storage management complexities.
- Search and Discovery Difficulties: Without proper organization, finding relevant information within massive unstructured data repositories becomes increasingly difficult, leading to duplicated efforts and missed insights.
- Lifecycle Management Complexity: Organizations struggle to implement effective policies for retention, archiving, and deletion of unstructured data, creating both compliance risks and unnecessary storage costs.
- Security and Governance Gaps: Unstructured data often contains sensitive information that remains unclassified and unprotected, creating significant security vulnerabilities and compliance gaps.
As one senior data architect at a Fortune 500 financial services firm described it: "Our structured data is like a well-organized library with a detailed catalog. Our unstructured data is more like thousands of filing cabinets filled with unlabeled folders scattered across hundreds of storage rooms. We know there's value in there, but finding and leveraging it is incredibly challenging."
Strategic Approaches
Forward-thinking organizations are adopting several strategies to address unstructured data challenges:
1. Automated Data Classification and Tagging
Modern AI-powered tools can automatically analyze, classify, and tag unstructured data at scale, making it more discoverable and manageable. These tools use natural language processing, computer vision, and machine learning to identify content types, extract key entities and concepts, and apply appropriate metadata.
A telecommunications company implemented an AI-driven classification system that automatically analyzed and tagged more than 50 million customer interaction records, including call transcripts, chat logs, and emails. This initiative improved customer service response times by 35% by making relevant historical interactions immediately accessible to service representatives.
2. Intelligent Data Tiering and Lifecycle Management
Organizations are implementing intelligent tiering strategies that automatically move data between storage tiers based on access patterns, age, and business value. This approach optimizes storage costs while ensuring appropriate accessibility.
"Organizations that implement automated lifecycle management for unstructured data typically reduce storage costs by 30-60% while improving compliance and data accessibility," notes a 2025 data management trends report.
3. Content-Aware Security and Governance
Advanced tools now enable organizations to automatically identify sensitive information within unstructured data and apply appropriate protection measures. These systems can detect personally identifiable information (PII), financial data, intellectual property, and other sensitive content, applying encryption, access controls, and data loss prevention measures accordingly.
A healthcare organization implemented content-aware security for its unstructured data repositories, automatically identifying and protecting patient information across more than 15 million documents and images. This initiative not only improved HIPAA compliance but also enabled broader, secure use of this data for research and analytics.
Challenge 2: Data Governance - From Control to Enablement
The Challenge
Traditional approaches to data governance have often focused primarily on control and compliance, emphasizing policies, procedures, and restrictions. While these aspects remain important, organizations now recognize that effective governance must also enable and accelerate data-driven innovation.
Key governance challenges include:
- Balancing Control and Access: Organizations struggle to strike the right balance between protecting sensitive data and making it accessible for analytical use.
- Distributed Governance Responsibilities: As data usage expands across the organization, centralized governance models become increasingly ineffective.
- Keeping Pace with Regulatory Changes: The rapidly evolving regulatory landscape creates ongoing compliance challenges.
- Measuring Governance Effectiveness: Many organizations lack clear metrics to evaluate whether their governance initiatives are effective.
"The most significant shift in data governance is the recognition that it must serve as an enabler of innovation rather than just a control function," explains a Chief Data Officer at a global manufacturing company. "Effective governance should make it easier for people to find, understand, trust, and use data appropriately—not just prevent misuse."
Strategic Approaches
Leading organizations are transforming their approach to data governance:
1. Federated Governance Models
Recognizing the limitations of centralized governance, many organizations are adopting federated models that distribute governance responsibilities across the organization while maintaining central oversight.
"Federated governance is the 'Goldilocks' solution," notes Jay Limburn, Chief Product Officer at Ataccama. "It strikes the perfect balance: a centralized team sets up the technology foundation, processes, and guardrails, while business teams retain their own data experts to manage domain-specific needs."
This collaborative approach—structured but flexible—ensures governance efforts are scalable, auditable, and still deeply connected to the business.
2. Governance as an Enabler
Forward-thinking organizations are reframing governance as an enabler of data-driven innovation rather than a set of restrictions. They're implementing self-service data access platforms with embedded governance controls, making it easier for users to find and use data appropriately.
A global consumer products company transformed its governance approach to focus on enablement, creating a self-service data platform with embedded controls. This shift reduced the time required to access data for analytics projects from weeks to hours while improving policy compliance.
3. Continuous Governance Evolution
Organizations are adopting more agile, iterative approaches to governance that can adapt to changing business needs and regulatory requirements.
"Data governance must be treated as a continuous process, not a one-time project," advises a data governance expert. "Organizations need governance models that can evolve as data sources, analytical techniques, and regulatory requirements change."
Challenge 3: Real-time Analytics - Shrinking the Insight Gap
The Challenge
In today's fast-paced business environment, the value of data often diminishes rapidly with time. Organizations increasingly need real-time or near-real-time insights to respond to changing market conditions, customer behaviors, and operational events.
Key challenges in real-time analytics include:
- Infrastructure Limitations: Traditional data architectures are often not designed for real-time processing of large data volumes.
- Data Integration Complexity: Real-time analytics typically requires integrating data from multiple sources, often with different formats and update frequencies.
- Analytical Complexity: Real-time analysis requires different approaches and algorithms compared to batch processing.
- Skills Gap: Many organizations lack personnel with experience in real-time analytics technologies and techniques.
"In 2025, we will start to see business intelligence and analytics tools shift from overwhelming, static dashboards to enabling dynamic, real-time 'conversations with data'," predicts Krishna Cheriath, CDAO at Thermo Fisher Scientific and Ataccama Strategic Advisor.
Strategic Approaches
Leading organizations are implementing several strategies to enable effective real-time analytics:
1. Modern Event-Driven Architectures
Organizations are adopting event-driven architectures that can process data as it's generated, enabling real-time insights and actions. These architectures typically include technologies such as stream processing platforms, in-memory databases, and message queues.
A major retailer implemented an event-driven architecture that processes more than 500 million events daily from online and in-store systems, enabling real-time inventory management, personalized customer experiences, and dynamic pricing. This initiative increased online conversion rates by 23% and reduced stockouts by 45%.
2. Intelligent Data Integration
Advanced data integration platforms now leverage AI to automate many aspects of data integration, including schema mapping, transformation logic, and data quality validation. These capabilities significantly reduce the time and expertise required to integrate diverse data sources.
"Organizations are moving beyond traditional ETL processes to more flexible, AI-assisted integration approaches that can adapt to changing data sources and analytical requirements," notes a data integration specialist.
3. Democratized Real-time Analytics
Organizations are deploying tools that make real-time analytics accessible to business users, not just data scientists. These platforms combine intuitive interfaces with powerful analytical capabilities, enabling a broader range of users to leverage real-time insights.
"Organizations will embrace AI-powered platforms that allow users to ask natural language questions and receive contextual insights, trends, and actionable recommendations instantly," predicts Cheriath. "This evolution will democratize data access, reduce decision fatigue, and make analytics more intuitive and impactful for all stakeholders."
Challenge 4: Data Product Operating Models - Products vs. Projects
The Challenge
Traditional approaches to data management have often treated data as a byproduct of business operations or as projects with defined endpoints. Forward-thinking organizations now recognize that treating data as a product—with ongoing management, enhancement, and support—creates significantly more value.
Key challenges in establishing data product operating models include:
- Organizational Structure: Traditional functional silos often impede the cross-functional collaboration needed for effective data products.
- Skills and Roles: Many organizations lack clear definition of the roles needed to support data products, such as data product owners and data engineers.
- Funding Models: Project-based funding models don't align well with the ongoing nature of data product management.
- Value Measurement: Organizations struggle to measure and communicate the value created by data products.
"The key to leveraging these advanced approaches requires a stable and trusted foundation, which more organizations will begin to focus on this year," says Akhil Lalwani, Former Chief Data Officer at Convex Insurance and Ataccama Strategic Advisor.
Strategic Approaches
Leading organizations are implementing several strategies to establish effective data product operating models:
1. Product-Oriented Teams and Roles
Organizations are establishing dedicated teams responsible for managing data products throughout their lifecycle. These teams typically include roles such as data product owner, data engineers, and quality assurance specialists.
"The first new role is that of a data product owner," explains Michael Meyer of Alation. "This role is very similar to a product manager role for software development, except for data... the data product owner is accountable to the business partners."
A global pharmaceutical company reorganized its analytics function around data products, establishing cross-functional teams responsible for key data domains like clinical trials, patient outcomes, and market intelligence. This shift reduced time-to-insight for new analytics initiatives by more than 60% and significantly improved data quality and consistency.
2. Data Marketplaces and Catalogs
Organizations are implementing internal data marketplaces that make data products discoverable and accessible to potential consumers. These platforms typically include rich metadata, usage examples, and self-service access capabilities.
"Similar to e-commerce platforms (like Amazon), data consumers should be able to find relevant data products in the marketplace effortlessly," advises Meyer. "Organizing products by business domains and including precise metadata enhances discoverability."
A financial services firm established an internal data marketplace that now includes more than 500 data products used by over 15,000 employees. This initiative reduced the time required to find and access relevant data by 85% and enabled more than 200 new analytical use cases in its first year.
3. Value-Based Metrics and Feedback Loops
Organizations are implementing metrics and feedback mechanisms that focus on the business value created by data products, not just technical metrics like volume or performance.
"Every data product must deliver clear business value," emphasizes Meyer. "Defining value starts with business stakeholders and their strategic objectives, ensuring data products directly contribute to key initiatives."
By implementing continuous feedback loops, organizations can ensure that data products evolve to meet changing business needs and create increasing value over time.
The AI Connection: Setting the Foundation for Intelligent Systems
The challenges of data management and analytics have taken on new urgency with the rapid adoption of artificial intelligence. As Ravi Ithal, GVP and CTO of Proofpoint DSPM notes: "For AI to deliver safe and reliable results, data teams must classify data properly before feeding it to those hungry LLMs."
This statement highlights a critical reality: the effectiveness of AI systems is fundamentally dependent on the quality, organization, and governance of the underlying data. Organizations that address the data challenges discussed in this article are not only improving their current analytical capabilities but also establishing the foundation for effective AI adoption.
Several key connections between data management and AI effectiveness include:
- Data Quality and AI Performance: AI systems trained on high-quality, well-governed data produce more accurate, reliable results than those trained on poor-quality data.
- Data Integration and AI Scope: The ability to integrate diverse data sources expands the scope and capability of AI systems, enabling more comprehensive insights and predictions.
- Real-time Data Processing and AI Responsiveness: Real-time data processing capabilities enable AI systems to respond dynamically to changing conditions, significantly enhancing their value.
- Data Products and AI Adoption: Well-designed data products make it easier to develop, deploy, and maintain AI systems, accelerating adoption and value creation.
"Investment in generative AI will move from experimentation to customer-impacting outcomes," predicts Lalwani. "A number of organizations have spent the last 18-24 months experimenting with generative AI and will now start to narrow down the specific areas in which this technology can make a significant impact."
Building Your Data Management Strategy: Key Recommendations
Based on the challenges and strategies discussed, here are key recommendations for technology leaders developing their data management and analytics strategies:
1. Assess Your Current State
Begin by assessing your organization's current capabilities across the four challenge areas:
- How effectively are you managing unstructured data growth?
- Is your governance approach enabling or hindering data-driven innovation?
- What is your capability for real-time analytics?
- To what extent do you manage data as products versus projects?
This assessment will help identify the most critical gaps and prioritize improvement initiatives.
2. Develop a Comprehensive Data Strategy
Based on your assessment, develop a data strategy that addresses all four challenge areas while aligning with your organization's broader business objectives. This strategy should include:
- Clear goals and metrics for each challenge area
- Specific initiatives to address identified gaps
- Required resources and investments
- Governance structures and processes
- Implementation roadmap and timeline
3. Invest in the Right Technologies
Select and implement technologies that address your specific challenges while providing flexibility for future needs. Key technology categories to consider include:
- Unstructured Data Management: Content analysis, classification, and lifecycle management tools
- Data Governance: Metadata management, data cataloging, and policy enforcement platforms
- Real-time Analytics: Event processing, streaming analytics, and in-memory computing solutions
- Data Product Management: Data marketplaces, data quality, and data observability tools
4. Build the Right Team and Skills
Develop the organizational capabilities needed to execute your data strategy effectively:
- Identify and define key roles, such as data product owners, data engineers, and data governance specialists
- Develop clear career paths and competency models for data-related roles
- Implement training and knowledge-sharing programs to build capabilities
- Consider strategic use of external partners to supplement internal capabilities
5. Start Small, Scale Fast
Begin with focused initiatives that deliver tangible value while building capabilities:
- Select high-value use cases that align with business priorities
- Implement pilot projects to demonstrate value and build momentum
- Document and share successes to build organizational support
- Scale successful approaches across the organization
6. Continuously Measure and Improve
Establish metrics and feedback mechanisms to track progress and guide continuous improvement:
- Define and track key performance indicators for each challenge area
- Implement regular reviews of progress and results
- Gather feedback from data producers and consumers
- Adjust strategies and initiatives based on results and feedback
Conclusion: From Data Management to Strategic Advantage
The data management and analytics challenges facing organizations in 2025 are substantial, but they also represent significant opportunities. Organizations that effectively address these challenges can transform data from a complex management problem into a strategic asset that drives innovation, operational excellence, and competitive advantage.
By implementing the strategies and recommendations outlined in this article, technology leaders can position their organizations to not only manage the data tsunami but to harness its power to drive business success. The organizations that master these challenges will be the ones that thrive in the increasingly data-driven business landscape of 2025 and beyond.
"The companies that succeed will be the ones that embrace these trends thoughtfully and use them to create real business value," notes a data management expert. "Data management is no longer just about keeping up—it's about staying ahead."
Join the Conversation
How is your organization addressing the challenges of unstructured data growth, governance, real-time analytics, and data product management? What strategies have you found most effective? Share your experiences and insights in the comments below to continue this important discussion.