Mastering the Cloud in 2025: How Leading Organizations Are Revolutionizing Their Digital Infrastructure
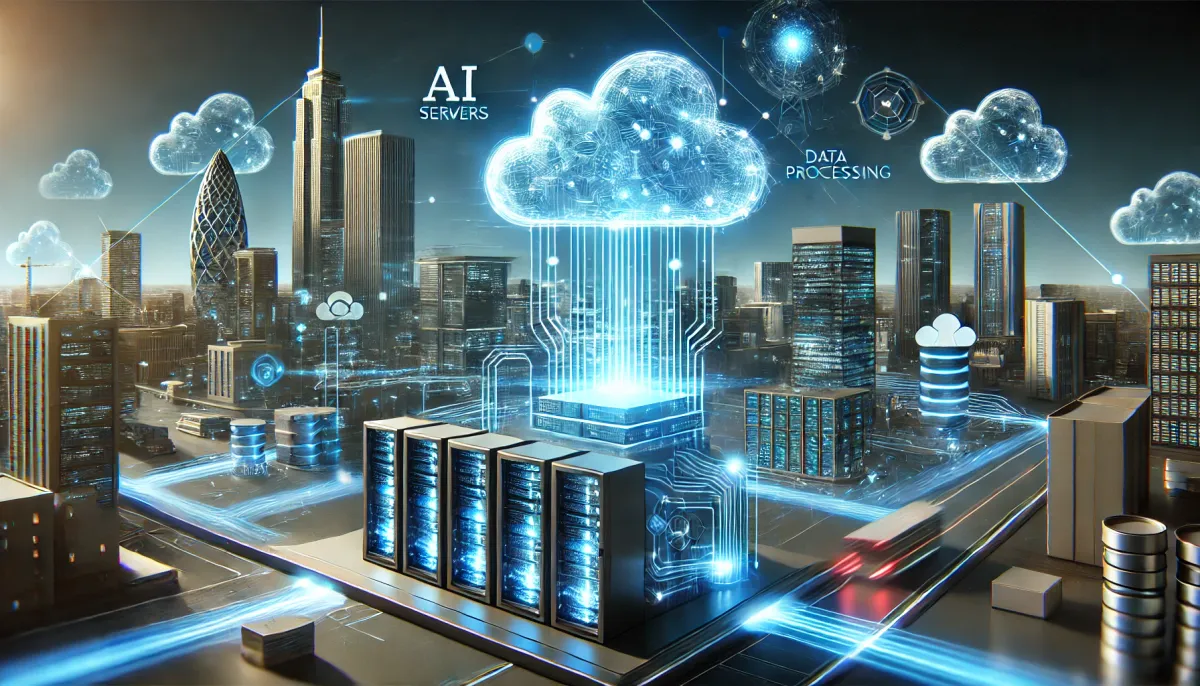
Introduction: The Shifting Paradigm of Cloud Computing
Did you know that by 2025, enterprise AI workloads are projected to consume more than 30% of total cloud infrastructure capacity? This staggering statistic from Gartner underscores a fundamental shift in how organizations are approaching their cloud strategies. As we navigate through 2025, the cloud landscape is undergoing a profound evolution, moving far beyond the simple lift-and-shift migrations of the past decade.
For technology leaders at the helm of enterprise technology decisions, understanding this evolution isn't just beneficial—it's imperative. With worldwide public cloud end-user spending forecasted to reach $723.4 billion in 2025, up from $595.7 billion in 2024, the stakes have never been higher.
In this article, we'll explore how cloud infrastructure and services are evolving to meet the complex demands of today's digital enterprises, with a particular focus on multi-cloud approaches, cost optimization strategies, data security compliance frameworks, and infrastructure requirements for AI workloads. By the end, you'll have gained actionable insights to refine your cloud strategy and position your organization for success in an increasingly cloud-powered world.
The Maturation of Cloud Strategies: Beyond Simple Adoption
The Rise of Multi-cloud and Hybrid Approaches
The days of single-cloud environments are rapidly fading into the past. According to Rackspace Technology's 2025 State of Cloud Report, organizations are increasingly shifting away from single-cloud approaches toward more flexible hybrid environments. The survey found that over 90% of organizations plan to make significant changes to their cloud strategy over the next two years, with 48% stating that a hybrid cloud for multi-environment deployment will be a key focus.
Why the shift? Multi-cloud and hybrid approaches offer a delicate balance between flexibility and control that single-cloud environments simply cannot match. Here's how this balance manifests in practice:
- Strategic Workload Placement: Different workloads have different requirements. A hybrid approach allows organizations to place workloads in environments that best suit their needs—whether that's public cloud for scalable applications, private cloud for sensitive data processing, or on-premises for legacy systems with specific compliance requirements.
- Vendor Leverage: By distributing workloads across multiple cloud providers, organizations gain negotiating power and reduce the risk of vendor lock-in. This distributes risk and creates competitive pressure among providers to deliver better services at more competitive rates.
- Geographical Flexibility: Multi-cloud deployments enable organizations to leverage region-specific cloud infrastructures, reducing latency for global applications and addressing data sovereignty requirements across different jurisdictions.
- Resilience and Business Continuity: Distributing workloads across multiple environments creates natural redundancy, enhancing disaster recovery capabilities and strengthening overall business continuity posture.
However, these benefits come with increased complexity. Ben Blanquera, Vice President of Technology and Sustainability at Rackspace, notes: "As organizations continue to advance their cloud infrastructure and leverage more complex environments such as hybrid and multicloud, the demand for skilled professionals is outstripping the supply."
This skills gap represents one of the most significant challenges in implementing effective multi-cloud strategies, necessitating investments in both talent acquisition and training programs to build internal expertise.
Cost Optimization: The Financial Discipline of Cloud Computing
As cloud environments grow more complex, so too does the challenge of managing costs. The initial promise of cloud computing—lower capital expenditure and pay-as-you-go models—has given way to the reality of complex billing structures and the potential for significant cost overruns without proper governance.
Enter FinOps (Financial Operations), a framework that has rapidly evolved from a niche practice to a mainstream discipline. FinOps enables organizations to establish financial accountability in variable cloud spending environments, driving informed decisions based on business value and actual cloud usage.
Key FinOps practices that are gaining traction in 2025 include:
- Real-time Cost Visibility: Implementing dashboards and monitoring tools that provide stakeholders with immediate visibility into cloud spending, broken down by project, department, and service.
- Automated Resource Optimization: Leveraging AI-driven tools to identify and eliminate unused or underutilized resources, right-size instances, and optimize storage tiers automatically.
- Reserved Capacity Management: Strategically purchasing reserved instances or savings plans to leverage discounts for predictable workloads while maintaining flexibility for variable demand.
- Chargeback and Showback Models: Implementing systems that attribute cloud costs to specific business units or projects, fostering accountability and encouraging more efficient resource utilization.
According to research from CloudEagle.ai, businesses now expect FinOps to not only optimize cloud costs but also drive key business outcomes through metrics like unit economics. This evolution represents a significant shift, positioning FinOps as a strategic business function rather than merely a cost-cutting initiative.
As one CIO of a Fortune 500 manufacturing company put it: "We've moved beyond viewing cloud cost optimization as a simple expense reduction exercise. Today, it's about aligning our cloud investments with business value delivery and ensuring every dollar spent is driving measurable outcomes."
Data Security and Compliance: Navigating an Increasingly Complex Landscape
As cloud adoption accelerates, so too does the regulatory landscape surrounding data security and privacy. For technology leaders, maintaining robust security measures across increasingly distributed cloud environments has become one of the most challenging aspects of cloud governance.
Data from Rackspace's 2025 State of Cloud Report reveals that an overwhelming 96% of organizations surveyed have established procedures and policies for data privacy and compliance in cloud environments. This near-universal adoption reflects the business-critical nature of cloud security and compliance in today's digital ecosystem.
Key trends shaping cloud security and compliance in 2025 include:
- Zero Trust Architecture: Moving beyond perimeter-based security to adopting a "never trust, always verify" approach that authenticates and authorizes every access request regardless of origin.
- AI-Powered Security Operations: Leveraging artificial intelligence to detect anomalies, identify potential threats, and automate response procedures across complex multi-cloud environments.
- Automated Compliance Frameworks: Implementing continuous compliance monitoring tools that automatically assess cloud resources against regulatory requirements, reducing the manual burden of compliance management.
- Data Sovereignty Solutions: Deploying region-specific cloud resources and implementing data residency controls to address the growing patchwork of data protection regulations globally.
According to Cyble's 2025 Cloud Security Guide, non-compliance can result in an average loss of $14.82 million annually for organizations, emphasizing the financial imperative of robust cloud security and compliance frameworks.
Piotr Kupisiewicz, CTO at Elisity, observes that "heavily regulated sectors like banking and government will treat new and existing AI tools as 'high-risk,' requiring strict compliance and security insurances." This trend is driving the adoption of microsegmentation and other advanced security practices that enable more granular control over cloud resources and data flows.
AI Workload Support: Scaling Infrastructure for Intelligent Applications
Perhaps the most transformative trend in cloud computing is the integration of artificial intelligence workloads into mainstream cloud environments. As organizations move beyond experimental AI projects to enterprise-scale deployments, cloud infrastructure must evolve to support the unique requirements of AI workloads.
Gartner projects that enterprise AI workloads will consume more than 30% of total cloud infrastructure capacity by 2025, a significant increase from previous years. This growth is driving several important infrastructure adaptations:
- Specialized Hardware Acceleration: Cloud providers are increasingly offering access to specialized hardware like GPUs, TPUs, and custom AI accelerators to support compute-intensive model training and inference workloads.
- High-Performance Storage Solutions: AI workloads, particularly those involving large language models or computer vision applications, require high-throughput, low-latency storage solutions that can efficiently feed data to compute resources.
- Elastic ML Infrastructure: Organizations are implementing elastic infrastructure that can dynamically scale to accommodate the bursty nature of AI workloads, with significant compute requirements during training phases and variable demand during inference.
- MLOps Platforms: Cloud-native machine learning operations platforms are becoming essential for managing the full lifecycle of AI models, from development and training to deployment and monitoring.
According to Rackspace's 2025 State of Cloud Report, 84% of organizations are actively integrating AI with their cloud strategies. When asked about the business outcomes driving this integration, 49% reported enhanced operational efficiency as their primary goal, while 45% cited improved data analytics insights.
One particularly noteworthy trend is the repatriation of certain AI workloads from public cloud to private cloud or on-premises infrastructure. Ben Blanquera of Rackspace notes that "nearly 80% of IT leaders at organizations that had repatriated workloads back to the private cloud or on-premises infrastructure were satisfied with the success of these moves." This suggests that while public cloud remains a valuable component of AI infrastructure strategies, organizations are taking a more nuanced approach based on performance, cost, and data governance considerations.
The Cloud-Powered Digital Core: Unlocking Advanced Intelligence
As we navigate through these evolving trends, it's worth considering the broader strategic context. Andy Tay, global lead of Accenture Cloud First, aptly summarizes the opportunity: "With a cloud-powered digital core in place, organizations can unlock advanced intelligence, industry-specific cloud innovations, enterprise efficiency and agility, and integrate new technologies, such as AI-enabled decision-making."
This concept of the "cloud-powered digital core" represents a mature understanding of cloud technology's role in enterprise architecture. Rather than viewing cloud as merely an infrastructure choice, forward-thinking organizations are positioning it as the foundational platform that enables broader digital transformation initiatives.
This perspective aligns with World Wide Technology's recommended cloud priorities for 2025, which emphasize harnessing "the power of intelligent cloud infrastructure and services for AI" to "rapidly deploy and scale innovative AI solutions and capabilities that drive business outcomes by taking advantage of the on-demand and elastic characteristics inherent to cloud."
Real-Life Case Studies: Cloud Evolution in Action
Case Study 1: Global Financial Services Firm Embraces Multi-Cloud Strategy
A leading global financial services institution with operations in over 50 countries faced significant challenges with their legacy cloud approach. Their single-provider strategy was creating concerns around cost escalation, regional performance issues, and potential regulatory complications in markets with strict data sovereignty requirements.
In response, the firm implemented a strategic multi-cloud architecture leveraging AWS for customer-facing applications, Microsoft Azure for data analytics workloads, and a private cloud infrastructure for sensitive financial data processing. The organization also implemented a central cloud management platform to provide unified governance across these environments.
Results:
- 27% reduction in overall cloud spending through optimized workload placement
- Improved application performance in regional markets by leveraging geographically distributed cloud resources
- Enhanced compliance posture with the ability to address varying regulatory requirements across different jurisdictions
- 40% faster time-to-market for new services through standardized deployment pipelines across cloud environments
Case Study 2: Manufacturing Enterprise Optimizes Cloud Costs Through FinOps
A multinational manufacturing company with annual cloud spending exceeding $25 million was experiencing rapid cost escalation without proportional business value delivery. Budget overruns had become common, creating tension between IT and business stakeholders.
The organization implemented a comprehensive FinOps program that included real-time cost visibility dashboards, chargeback mechanisms, and AI-driven resource optimization. Cross-functional FinOps teams with representatives from IT, finance, and business units were established to drive shared accountability.
Results:
- Reduced cloud waste by 35% in the first six months through elimination of zombie resources and right-sizing
- Improved forecasting accuracy from ±30% to ±5%, enabling more precise budgeting
- Established clear cost attribution to business initiatives, shifting conversations from IT spending to business value
- Developed unit economics metrics that tied cloud costs directly to business outcomes (e.g., cost per transaction, cost per customer)
Case Study 3: Healthcare Provider Scales AI Infrastructure for Diagnostic Imaging
A large healthcare system sought to implement an AI-powered diagnostic imaging solution to improve radiology workflow efficiency and diagnostic accuracy. Initial prototypes showed promising results, but scaling the solution to production volumes created significant infrastructure challenges.
The organization implemented a hybrid AI infrastructure strategy, leveraging public cloud resources for model training and development while deploying inference workloads on private cloud infrastructure connected directly to their clinical systems. An MLOps platform was implemented to manage model versioning, validation, and deployment across environments.
Results:
- Successfully scaled to processing over 2,000 diagnostic images daily with average inference times under 3 minutes
- Reduced diagnostic waiting times by 40% while improving detection rates for certain conditions by up to 28%
- Maintained full compliance with healthcare data protection regulations through careful data governance
- Achieved 99.9% infrastructure availability for this mission-critical application
Actionable Tips for Cloud Evolution Success
- Conduct a Workload Placement Assessment: Systematically evaluate your application portfolio to determine the optimal environment (public cloud, private cloud, or on-premises) for each workload based on performance, security, compliance, and cost considerations.
- Implement Cloud Financial Management Early: Don't wait for cloud costs to become a problem. Establish FinOps practices from the beginning of your cloud journey, focusing on visibility, accountability, and optimization as foundational capabilities.
- Develop a Unified Security and Compliance Framework: Create a consistent security model that spans all your cloud environments, with centralized policy management, monitoring, and automated compliance validation.
- Build Cloud Centers of Excellence: Establish cross-functional teams that bring together expertise in cloud architecture, security, operations, and financial management to guide your organization's cloud evolution and share best practices.
- Invest in Cloud Skill Development: Address the skills gap through a combination of targeted hiring, training programs, and partnerships with managed service providers to ensure your team can effectively manage increasingly complex cloud environments.
- Create an AI Infrastructure Roadmap: Develop a forward-looking plan for how your cloud infrastructure will evolve to support growing AI workloads, including considerations for specialized hardware, high-performance storage, and MLOps capabilities.
- Implement Automated Governance: Deploy tools that can automatically enforce policies for resource provisioning, security, compliance, and cost management across all your cloud environments.
- Establish Cloud Value Metrics: Move beyond simple cost tracking to develop metrics that connect cloud investments to business outcomes, enabling more strategic conversations about cloud spending and priorities.
Common FAQs About Cloud Infrastructure Evolution
Q1: How do we determine which workloads should remain on-premises versus moving to public or private cloud?
A: Workload placement decisions should be based on a systematic assessment of several factors: performance requirements, data sensitivity, regulatory compliance needs, integration with other systems, and total cost of ownership. Many organizations are implementing formal methodologies such as the AWS Cloud Adoption Framework or Microsoft's Cloud Journey Assessment to guide these decisions. Generally, workloads with strict latency requirements, heavy regulatory constraints, or significant investments in specialized hardware may be better suited for on-premises or private cloud environments.
Q2: How can we effectively manage costs across multiple cloud providers?
A: Managing multi-cloud costs requires both technological solutions and organizational practices. Implement a cloud management platform that provides unified visibility across providers, standardize tagging schemes for consistent resource attribution, leverage provider-specific optimization tools while implementing vendor-neutral policies, and establish clear accountability through chargeback or showback mechanisms. Most importantly, develop FinOps capabilities that bring together finance, technology, and business stakeholders to make cost-informed decisions.
Q3: What are the most important security considerations for multi-cloud environments?
A: The primary considerations include: implementing consistent identity and access management across all environments, establishing unified security policies with environment-specific implementations, deploying centralized monitoring and logging to maintain visibility, ensuring data encryption both in transit and at rest across all environments, developing cross-cloud incident response procedures, and maintaining compliance validation tools that work across providers. Many organizations are adopting Cloud Security Posture Management (CSPM) platforms to help address these challenges in a more automated and consistent manner.
Q4: How should we adapt our infrastructure to support growing AI workloads?
A: Start by understanding the different phases of AI workloads (development, training, and inference) and their unique requirements. Develop capabilities to provide elastic access to specialized hardware like GPUs, implement high-performance storage solutions optimized for AI data pipelines, ensure your networking infrastructure can handle the data volumes, and build MLOps practices that bridge the gap between data science and IT operations. Consider hybrid approaches that leverage public cloud for development and training while deploying inference workloads closer to data sources.
Conclusion: Embracing the Next Wave of Cloud Evolution
As we've explored throughout this article, cloud infrastructure and services are evolving rapidly to meet the changing needs of digital enterprises. The shift toward multi-cloud and hybrid approaches, the maturation of cloud financial management practices, the increasing complexity of security and compliance requirements, and the growing importance of AI-ready infrastructure are collectively reshaping how organizations approach their cloud strategies.
For technology leaders navigating this evolution, the key to success lies in viewing cloud not merely as an infrastructure choice but as a foundational platform for broader digital transformation. As Andy Tay of Accenture aptly noted, a well-designed cloud-powered digital core enables organizations to unlock advanced intelligence, drive innovation, and integrate transformative technologies like AI.
By thoughtfully addressing the strategic considerations outlined in this article and learning from the experiences of organizations that have successfully navigated similar journeys, you can position your enterprise to fully realize the benefits of modern cloud infrastructure—enhanced agility, optimized costs, strengthened security, and the ability to harness the full potential of AI and other emerging technologies.
The question is no longer whether to embrace cloud, but how to evolve your cloud approach to drive maximum business value in an increasingly complex and competitive landscape. The organizations that answer this question effectively will be well-positioned to thrive in 2025 and beyond.
What steps is your organization taking to evolve its cloud infrastructure and services? We'd love to hear about your experiences and challenges in the comments below.