Beyond Hype to Results: Solving AI Implementation and ROI Challenges for Enterprise Leaders
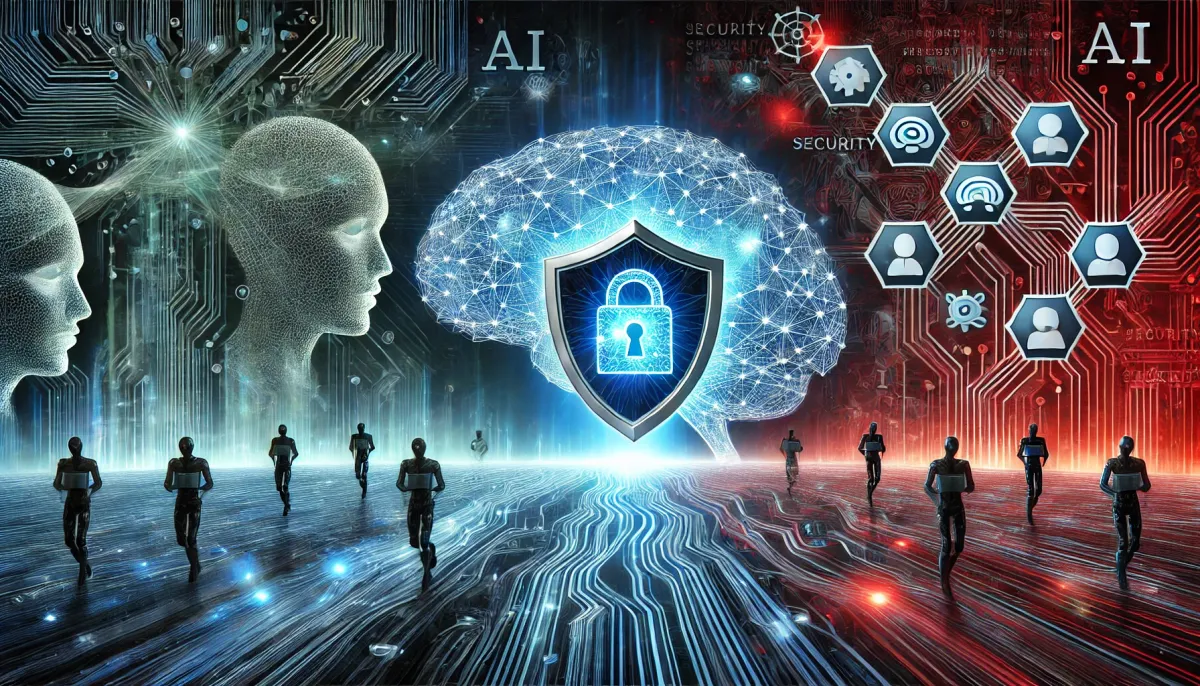
In the race to adopt AI, enterprise leaders face a critical challenge: demonstrating tangible returns on massive investments. As organizations pour hundreds of millions into AI initiatives, the pressure to show business value grows. This article explores the complex puzzle of AI ROI and provides actionable strategies for CTOs and CIOs to bridge the gap between AI potential and measurable business impact.
The AI Investment Paradox: Big Spending, Elusive Returns
In boardrooms across the globe, a familiar conversation unfolds: "We've invested millions in AI—where are the returns?" According to KPMG's recent survey, nearly 70% of enterprise leaders plan to spend between $50 million and $250 million on AI initiatives in 2025 alone. Yet only 31% expect to measure ROI within six months, and virtually none report having achieved meaningful returns yet.
The disconnect is stark. While AI promises transformative potential, the path from implementation to measurable value remains obscure for many organizations. As one CIO of a Fortune 500 company recently confided, "We've built impressive AI capabilities, but translating them into bottom-line impact has been more challenging than anticipated."
This challenge is particularly acute as we move from the experimentation phase to expectations of real-world value. As Bill Pappas, MetLife's head of global technology and operations, explains: "Technology investments, such as in generative AI, are a priority in addressing the need to meet rising expectations while also driving operational agility and resilience. In a time where trust and reliability are paramount, meeting these expectations through technology isn't just a differentiator—it's now a business imperative."
Why AI ROI Measurement Defies Traditional Approaches
Traditional ROI frameworks often fall short when applied to AI initiatives for several critical reasons:
1. Multi-dimensional Impact
Unlike conventional IT projects with clear-cut outcomes (e.g., reduced server costs), AI initiatives often generate value across multiple dimensions simultaneously. A customer service AI might reduce operational costs, increase employee productivity, improve customer satisfaction, and accelerate problem resolution—all at once. Which metric matters most?
2. Delayed Value Realization
While some AI applications deliver immediate results (like process automation), many others—particularly those focused on decision support, predictive capabilities, or innovation acceleration—may take months or years to demonstrate their full value. This temporal disconnect between investment and returns challenges traditional ROI timeframes.
3. Indirect Benefits
Some of AI's most significant impacts are indirect or difficult to quantify. How do you measure the value of improved decision quality, enhanced innovation capacity, or more agile responses to market changes? These benefits, while substantial, often escape traditional ROI calculations.
4. Moving Target Syndrome
In a rapidly evolving technology landscape, the goalposts for AI success constantly shift. What constituted impressive AI capabilities a year ago may be baseline expectations today, making comparative ROI analyses particularly challenging.
The Foundation of AI Success: Data Quality and Governance
According to KPMG's research, a staggering 85% of leaders cite data quality as their most significant challenge in AI strategies for 2025. This statistic underscores a fundamental truth: AI systems are only as good as the data that feeds them.
Poor data quality manifests in multiple ways:
- Inaccurate outputs and recommendations
- Biased decision-making
- Inconsistent performance across different business contexts
- Inability to scale solutions enterprise-wide
"For AI to deliver safe and reliable results, data teams must classify data properly before feeding it to those hungry LLMs," points out Ravi Ithal, GVP and CTO of Proofpoint DSPM.
The solution begins with robust data governance. Forward-thinking CTOs and CIOs are investing in:
- Comprehensive data quality frameworks
- Data standardization initiatives
- Master data management systems
- Data catalogs and lineage tracking
- Ethics-driven data governance policies
These investments, while not immediately visible on the AI ROI ledger, establish the foundation upon which successful AI initiatives are built.
From Experimentation to Value Creation: The Strategic Shift
As AI matures within enterprises, a strategic pivot is essential: from technology-driven experimentation to outcome-focused implementation. This shift requires aligning AI initiatives with core business objectives rather than pursuing AI for its own sake.
Successful organizations are approaching this challenge through three strategic lenses:
1. Business-First Use Case Selection
Rather than starting with the technology, begin with pressing business problems or opportunities where AI can deliver tangible value. This ensures alignment between AI capabilities and business priorities.
Case Study: A leading insurance company focused its AI efforts on claims processing—a high-volume, error-prone, and customer-critical function. By implementing AI to automate routine claims and flag complex ones for human review, they reduced processing time by 72% and improved accuracy by 35%, delivering clear, measurable ROI within four months.
2. Value-Chain Integration
Instead of creating standalone AI applications, focus on integrating AI capabilities into existing business processes and workflows. This approach reduces adoption barriers and makes value measurement more straightforward.
Case Study: A global manufacturing firm embedded predictive maintenance AI directly into existing equipment monitoring systems. This seamless integration drove 92% adoption among factory floor operators within two months and reduced unplanned downtime by 47%—a clear ROI metric tied directly to production efficiency and cost savings.
3. Composite Metrics Approach
Develop multidimensional ROI frameworks that capture AI's diverse impacts across efficiency, effectiveness, and innovation dimensions.
Example Framework:
- Efficiency Metrics: Cost reduction, time savings, resource optimization
- Effectiveness Metrics: Error reduction, quality improvement, customer satisfaction
- Innovation Metrics: New product development, market responsiveness, competitive differentiation
This balanced scorecard approach provides a more holistic view of AI's business impact than traditional financial metrics alone.
Technical Debt: The Hidden ROI Killer
While not immediately visible on balance sheets, technical debt represents one of the most significant threats to long-term AI ROI. Forrester predicts that 75% of technology leaders will face moderate to severe technical debt by 2026 as organizations rush to implement AI solutions.
This debt manifests in several ways:
- Fragmented AI platforms and tools
- Inconsistent integration approaches
- Shadow AI initiatives outside governance frameworks
- Insufficient documentation and knowledge transfer
- Security and compliance gaps
Each represents hidden costs that erode ROI over time. Forward-thinking CTOs are addressing these challenges through:
- Centralized AI governance frameworks
- Enterprise architecture standards for AI
- Balanced "build vs. buy" decision models
- Technical debt remediation programs
- AI-specific development and deployment standards
As Gary Bailey, CIO at Phillips Edison & Co. notes, "With AI agents on the horizon, there will be a significant number of business processes that will be a much better fit for AI than we have previously seen. We are in the midst of an extremely opportunistic window."
Democratization vs. Control: Finding the Balance
One of the most challenging aspects of AI implementation involves balancing democratization (making AI broadly available across the organization) with appropriate governance and control.
Too much centralization stifles innovation and adoption, while excessive democratization can lead to fragmentation, security risks, and wasted resources. Finding the right balance is critical to long-term AI ROI.
Leading organizations are addressing this challenge through:
1. AI Centers of Excellence (CoEs)
Establishing centralized teams responsible for standards, best practices, and shared AI services, while enabling business units to implement solutions within the established framework.
2. Self-Service AI Platforms with Guardrails
Providing business users with access to AI capabilities through governed platforms that enforce security, compliance, and quality standards automatically.
3. Federated Governance Models
Creating tiered governance approaches where risk levels determine the degree of centralized oversight required—higher-risk AI applications receive more scrutiny, while lower-risk use cases benefit from streamlined approval processes.
4. AI Education Initiatives
Developing comprehensive AI literacy programs to ensure business users understand both AI capabilities and associated risks, enabling more responsible democratization.
As Alan Thorogood, research leader at the MIT Center for Information Systems Research (CISR), advises: "The top priority for 2025 is to change your IT operating model to fit your organization's needs, which have surely changed recently."
Common FAQs About AI ROI
Q: How quickly should we expect to see ROI from our AI investments?
A: Timeframes vary significantly by use case. Process automation typically shows returns within 3-6 months, while more complex applications like predictive analytics or decision support may take 12-18 months or longer. Setting realistic expectations aligned with use case complexity is essential.
Q: What's the most effective approach to measuring AI ROI?
A: Use a balanced scorecard approach that captures impacts across efficiency (cost/time savings), effectiveness (quality improvements), and innovation dimensions (new capabilities). Focusing exclusively on cost reduction often understates AI's true business value.
Q: How can we accelerate AI value realization?
A: Focus on rapid iteration and learning cycles rather than perfect implementation. Start with high-impact, lower-complexity use cases that deliver quick wins, then build on that momentum. Ensure tight alignment between AI initiatives and core business priorities.
Q: What's the right organizational structure for AI implementation?
A: Most successful organizations employ a hybrid model—centralized governance and shared services combined with embedded AI capabilities within business units. This balances consistency and efficiency with business alignment and adoption.
Q: How do we address employee concerns about AI replacing jobs?
A: Frame AI as augmentation rather than replacement, focus on reskilling programs, and showcase how AI can eliminate routine tasks while creating space for higher-value work. Employee resistance can significantly undermine AI ROI if not proactively addressed.
The Path Forward: Strategic Priorities for 2025
As enterprises navigate the complex landscape of AI implementation and ROI, several strategic priorities emerge for forward-thinking CTOs and CIOs:
1. Align AI with Business Transformation
Position AI initiatives within broader digital transformation efforts rather than as standalone technology projects. This alignment ensures AI contributes to fundamental business objectives rather than representing technological capability for its own sake.
2. Invest in Data Foundation
Prioritize investments in data quality, integration, and governance as the bedrock of successful AI implementation. Without this foundation, even the most sophisticated AI capabilities will fail to deliver expected returns.
3. Develop AI-Specific ROI Frameworks
Create measurement approaches tailored to AI's unique characteristics, incorporating both quantitative and qualitative dimensions, short and long-term impacts, and direct and indirect benefits.
4. Address the Human Element
Recognize that AI adoption ultimately depends on human acceptance and integration. Invest in change management, training, and organizational alignment to maximize adoption and value realization.
5. Balance Innovation and Standardization
Create frameworks that enable experimentation and innovation while maintaining necessary standards and controls. This balanced approach prevents both stagnation and chaos in AI implementation.
Conclusion: From Investment to Impact
The journey from AI investment to measurable business impact isn't straightforward, but it's increasingly essential for competitive advantage. By addressing the foundational challenges of data quality, aligning AI initiatives with business priorities, adopting multidimensional ROI frameworks, and balancing governance with innovation, CTOs and CIOs can bridge the gap between AI's promise and its delivered value.
The organizations that master this transition—from AI as a technological experiment to AI as a business value generator—will distinguish themselves in the marketplace and justify the significant investments being made in these transformative technologies.
As we move deeper into 2025, the question is shifting from "Should we invest in AI?" to "How do we ensure our AI investments deliver measurable business impact?" By focusing on the strategies outlined in this article, enterprise leaders can confidently answer the latter question—and transform the AI investment paradox into a clear path to value creation.
What AI implementation or ROI measurement challenges is your organization facing? Share your experiences in the comments below, or reach out directly to discuss strategies for addressing these common obstacles.